In the world of data analysis, dealing with missing values is crucial for obtaining accurate results, and IBM SPSS is a powerful tool to address this challenge. Missing values can arise from various sources, including data entry errors, non-responses in surveys, or even data corruption. Understanding how to handle these missing values effectively can greatly enhance the integrity of your analysis and the insights you derive from your data.
This article delves into the concept of missing values in IBM SPSS, exploring their types, methods for handling them, and the implications of these methods on your analysis. We will also provide practical examples and tips to ensure you are equipped with the necessary knowledge to tackle missing data confidently.
By the end of this article, you will have a comprehensive understanding of IBM SPSS missing values, the tools available to address them, and best practices to ensure your analyses remain robust and reliable. Whether you are a beginner or an experienced SPSS user, this guide aims to enhance your expertise in managing missing data.
Table of Contents
- 1. Understanding Missing Values
- 2. Types of Missing Values in SPSS
- 3. Impact of Missing Values on Data Analysis
- 4. Handling Missing Values in IBM SPSS
- 5. Imputation Methods for Missing Values
- 6. Best Practices for Dealing with Missing Values
- 7. Real-World Examples of Missing Values Handling
- 8. Conclusion
1. Understanding Missing Values
Missing values refer to the absence of data points for certain variables within a dataset. In SPSS, missing values can pose significant challenges, as they can lead to biased results or reduced statistical power in analyses. Understanding the nature of missing data is essential to determine the best approach for handling it.
2. Types of Missing Values in SPSS
IBM SPSS recognizes various types of missing values, which can broadly be categorized into three groups:
- Missing Completely at Random (MCAR): The missing values are randomly distributed across all observations and do not depend on any variables.
- Missing at Random (MAR): The likelihood of a value being missing is related to observed data but not to the missing data itself.
- Not Missing at Random (NMAR): The missingness is related to the value of the missing data itself, making it challenging to handle appropriately.
3. Impact of Missing Values on Data Analysis
Missing values can significantly impact the outcome of statistical analyses. Some common consequences include:
- Reduced Sample Size: Analyses may be conducted on a smaller subset of data, leading to less reliable results.
- Biased Estimates: If the missing data is not handled properly, it can introduce bias into the results.
- Decreased Statistical Power: The overall ability to detect effects can be diminished due to missing values.
4. Handling Missing Values in IBM SPSS
IBM SPSS offers several strategies for handling missing values. Users can choose to:
- Exclude cases with missing values from the analysis (listwise deletion).
- Use pairwise deletion for correlation analyses.
- Implement various imputation methods to estimate missing values.
5. Imputation Methods for Missing Values
Imputation is a common method used to address missing values, with several approaches available in SPSS:
5.1 Mean/Median/Mode Imputation
This method involves replacing missing values with the mean, median, or mode of the observed data. While simple, it can introduce bias if the data is not MCAR.
5.2 Regression Imputation
Regression imputation predicts missing values based on the relationships between variables in the dataset, providing potentially more accurate estimates than mean imputation.
5.3 Multiple Imputation
This advanced technique involves creating multiple datasets with different imputations and combining the results to account for the uncertainty associated with the missing values.
6. Best Practices for Dealing with Missing Values
To effectively manage missing values in SPSS, consider the following best practices:
- Conduct exploratory data analysis to understand the pattern and extent of missing values.
- Choose the appropriate method for handling missing data based on its type and potential impact on analysis.
- Document all decisions made regarding missing values for transparency and reproducibility.
7. Real-World Examples of Missing Values Handling
To illustrate the importance of managing missing values, let's explore a couple of real-world scenarios:
- In a healthcare study, if patient follow-up data is missing due to non-responses, applying multiple imputation can help maintain the integrity of the dataset for analysis.
- In market research, missing survey responses can skew results; using regression imputation can help provide a more accurate picture of consumer behavior.
8. Conclusion
Handling missing values in IBM SPSS is a critical aspect of ensuring the reliability and validity of your data analysis. By understanding the types of missing values, their impact, and the various methods available for addressing them, you can enhance the quality of your research and insights. We encourage you to explore the tools SPSS offers, implement best practices, and share your experiences with the community.
If you found this article helpful, please leave a comment, share it with your colleagues, or read more articles on our site to further enhance your data analysis skills.
Thank you for taking the time to learn about IBM SPSS missing values. We hope to see you back here for more insightful content!


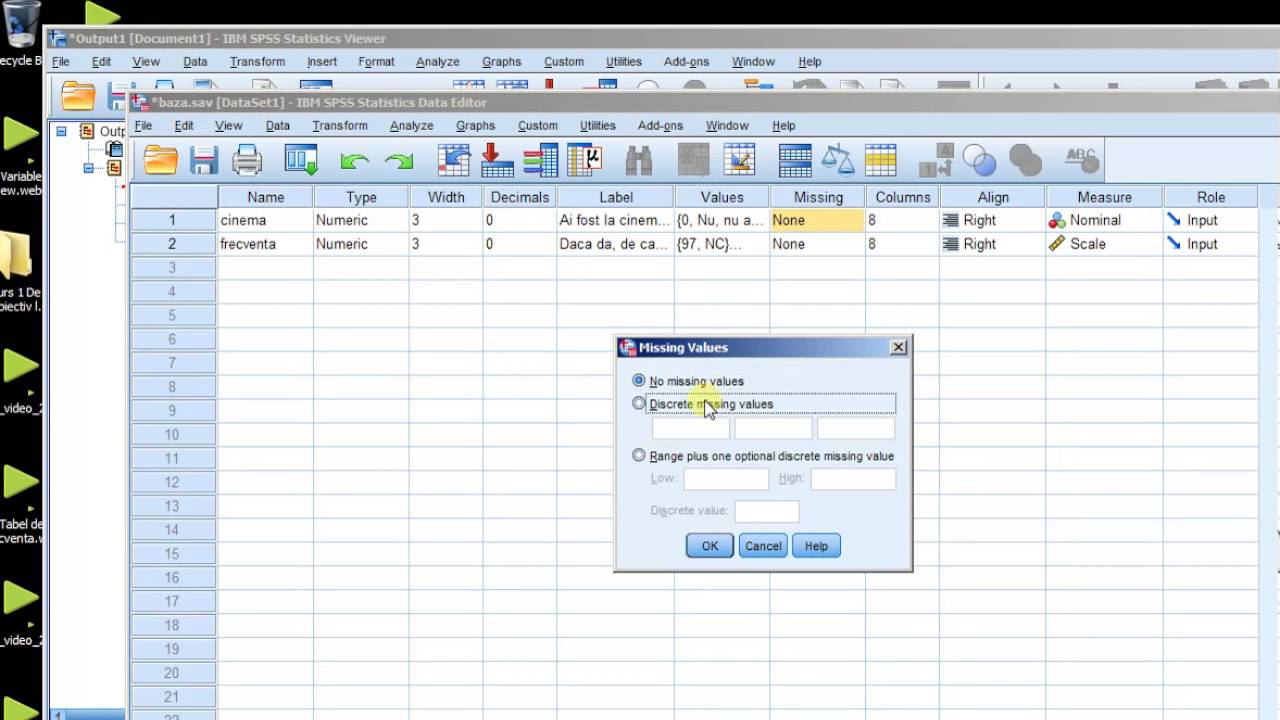